Quartile Calculator
Is this tool helpful?
How to Use the Quartile Calculator Effectively
Our Quartile Calculator is designed to help you quickly and accurately compute quartiles, median, and interquartile range for your dataset. Here’s a step-by-step guide on how to use this powerful tool:
1. Enter Your Data
In the “Enter Data” field, input your numeric values. You can separate the numbers using commas, spaces, or new lines. For example:
- 12, 5, 22, 30, 18
- 3.5 7.2 9.1 15.6 20.3
- 100
200
150
175
225
2. Choose the Calculation Method
Select your preferred calculation method from the dropdown menu. The options are:
- Inclusive: Includes the median when calculating Q1 and Q3
- Exclusive: Excludes the median when calculating Q1 and Q3
- Percentile: Uses percentile-based formulas for quartile calculations
3. Visualization Option
Check the “Include Visualization” box if you want to see a box plot representation of your data distribution.
4. Calculate
Click the “Calculate” button to process your data and view the results.
5. Interpret the Results
The calculator will display:
- First Quartile (Q1)
- Second Quartile (Median, Q2)
- Third Quartile (Q3)
- Interquartile Range (IQR)
If you selected the visualization option, you’ll also see a box plot graph below the numerical results.
Understanding Quartiles: Your Gateway to Data Distribution Insights
Quartiles are powerful statistical measures that divide a dataset into four equal parts, each containing 25% of the data. They provide crucial insights into the distribution and spread of your data, making them invaluable tools for data analysis, statistical inference, and decision-making across various fields.
The Power of Quartiles in Data Analysis
Quartiles offer a comprehensive view of your data’s central tendency and variability. Here’s what each quartile represents:
- First Quartile (Q1): The 25th percentile, below which 25% of the data falls
- Second Quartile (Q2 or Median): The 50th percentile, representing the middle value of the dataset
- Third Quartile (Q3): The 75th percentile, below which 75% of the data falls
The Interquartile Range (IQR), calculated as Q3 – Q1, measures the spread of the middle 50% of your data, providing a robust measure of variability that’s less sensitive to outliers than the range.
Mathematical Foundations of Quartile Calculations
The calculation of quartiles involves sorting the data and finding the appropriate position based on the dataset size. Here are the general formulas for quartile positions:
$$Q1 \text{ position} = \frac{n + 1}{4}$$
$$Q2 \text{ position} = \frac{n + 1}{2}$$
$$Q3 \text{ position} = \frac{3(n + 1)}{4}$$
Where n is the number of values in the dataset.
For datasets where these positions result in non-integer values, interpolation between adjacent values is used to determine the exact quartile values.
Benefits of Using the Quartile Calculator
1. Time-Saving Efficiency
Manual calculation of quartiles can be time-consuming, especially for large datasets. Our Quartile Calculator automates this process, providing instant results and allowing you to focus on data interpretation rather than computation.
2. Accuracy and Consistency
Human error is eliminated with our calculator, ensuring precise results every time. The tool consistently applies the chosen calculation method, providing reliable quartile values across different datasets.
3. Flexibility in Calculation Methods
With options for inclusive, exclusive, and percentile-based calculations, our tool adapts to various statistical standards and preferences, making it suitable for a wide range of applications and academic requirements.
4. Visual Representation
The optional box plot visualization offers an immediate graphical representation of your data distribution, enhancing understanding and facilitating quick insights into data spread and potential outliers.
5. Educational Value
For students and educators, this tool serves as an excellent learning aid, demonstrating the practical application of quartile calculations and data visualization techniques.
Addressing User Needs: Practical Applications of Quartile Analysis
Identifying Data Spread and Outliers
Quartiles help in understanding the spread of your data and identifying potential outliers. Let’s consider a dataset of exam scores:
45, 56, 62, 65, 70, 72, 75, 78, 80, 82, 85, 88, 90, 92, 95
Using our Quartile Calculator with the inclusive method, we get:
- Q1 (First Quartile): 65
- Q2 (Median): 78
- Q3 (Third Quartile): 88
- IQR (Interquartile Range): 23
This tells us that:
- 25% of students scored below 65
- 50% of students scored below 78
- 75% of students scored below 88
- The middle 50% of scores span a range of 23 points
To identify potential outliers, we can use the IQR method:
- Lower bound: Q1 – 1.5 * IQR = 65 – 1.5 * 23 = 30.5
- Upper bound: Q3 + 1.5 * IQR = 88 + 1.5 * 23 = 122.5
In this case, there are no outliers as all scores fall within these bounds.
Comparing Distributions
Quartiles are excellent for comparing distributions of different datasets. Let’s compare two classes’ exam scores:
Class A: 60, 65, 70, 72, 75, 78, 80, 82, 85, 88, 90
Class B: 55, 58, 62, 68, 70, 72, 75, 80, 85, 88, 92
Using our calculator for both datasets:
Class A results:
- Q1: 70
- Q2 (Median): 78
- Q3: 85
- IQR: 15
Class B results:
- Q1: 62
- Q2 (Median): 72
- Q3: 85
- IQR: 23
This comparison reveals that while both classes have similar high performers (Q3), Class A has a higher median and Q1, indicating better overall performance. The larger IQR in Class B suggests more variability in scores.
Real-World Applications of Quartile Analysis
1. Financial Analysis
In finance, quartiles are used to analyze stock performance, assess risk, and make investment decisions. For example, a fund manager might use quartile analysis to rank mutual funds based on their returns:
Annual Returns (%): 2.5, 3.1, 3.8, 4.2, 4.5, 5.0, 5.3, 5.7, 6.1, 6.5, 7.0, 7.8
Using our calculator:
- Q1: 3.8%
- Q2 (Median): 5.15%
- Q3: 6.2%
- IQR: 2.4%
This analysis helps identify top-performing funds (above Q3) and underperforming funds (below Q1), guiding investment strategies and portfolio allocations.
2. Human Resources and Salary Analysis
HR departments use quartile analysis to establish salary ranges and ensure fair compensation. Consider this dataset of annual salaries (in thousands) for a specific role:
45, 48, 50, 52, 55, 58, 60, 62, 65, 68, 70, 75, 80, 85, 90
Quartile analysis results:
- Q1: $52,000
- Q2 (Median): $62,000
- Q3: $70,000
- IQR: $18,000
This information helps HR professionals set competitive salary ranges, with Q1 potentially representing entry-level salaries, the median for mid-level positions, and Q3 for senior roles or top performers.
3. Quality Control in Manufacturing
Manufacturers use quartile analysis to monitor product quality and identify process improvements. For instance, analyzing the diameter of produced bolts (in mm):
9.8, 9.9, 10.0, 10.0, 10.1, 10.1, 10.2, 10.2, 10.2, 10.3, 10.3, 10.4
Quartile analysis:
- Q1: 10.0 mm
- Q2 (Median): 10.15 mm
- Q3: 10.3 mm
- IQR: 0.3 mm
This analysis helps in setting quality control limits and identifying when the manufacturing process may be drifting out of specification.
Frequently Asked Questions (FAQ)
Q1: What’s the difference between the inclusive and exclusive methods?
A1: The inclusive method includes the median value when calculating Q1 and Q3 for datasets with an odd number of values. The exclusive method always excludes the median. This can lead to slightly different results, especially for smaller datasets.
Q2: How many data points do I need for a meaningful quartile analysis?
A2: While quartiles can be calculated with as few as three data points, a larger dataset (preferably 20 or more) provides more reliable and meaningful results. The more data points, the more representative the quartiles will be of the true data distribution.
Q3: Can I use quartile analysis for non-numeric data?
A3: Quartile analysis is primarily designed for numeric data. For categorical or ordinal data, other methods like frequency analysis or median calculations might be more appropriate.
Q4: How do I interpret the box plot visualization?
A4: The box plot shows the minimum, Q1, median, Q3, and maximum values. The box represents the IQR, with the median line inside. Whiskers extend to the minimum and maximum values, and any points beyond the whiskers are potential outliers.
Q5: What’s the relationship between quartiles and percentiles?
A5: Quartiles are specific percentiles. Q1 is the 25th percentile, the median (Q2) is the 50th percentile, and Q3 is the 75th percentile. Our calculator’s percentile method uses this relationship for calculations.
Q6: Can quartile analysis help identify a normal distribution?
A6: While quartile analysis alone can’t definitively determine if a distribution is normal, it can provide clues. In a normal distribution, the median should be close to the mean, and the distance from Q1 to the median should be similar to the distance from the median to Q3.
Q7: How does the Interquartile Range (IQR) relate to standard deviation?
A7: Both IQR and standard deviation measure variability, but IQR is less sensitive to outliers. For a normal distribution, the IQR is approximately 1.35 times the standard deviation. However, IQR is more robust for non-normal distributions.
Q8: Can I use this calculator for very large datasets?
A8: Yes, our Quartile Calculator is designed to handle large datasets efficiently. However, for extremely large datasets (millions of data points), you might experience a slight delay in processing.
Q9: How do quartiles help in detecting outliers?
A9: Outliers are often defined as values below Q1 – 1.5 * IQR or above Q3 + 1.5 * IQR. This method, known as the IQR method, is less sensitive to extreme values than using standard deviations from the mean.
Q10: Can quartiles be used for data that isn’t normally distributed?
A10: Absolutely! One of the strengths of quartile analysis is that it doesn’t assume a normal distribution. It’s particularly useful for skewed distributions where measures like mean and standard deviation might be misleading.
By leveraging our Quartile Calculator and understanding these concepts, you’ll be well-equipped to gain valuable insights from your data, whether you’re a student, researcher, financial analyst, or business professional. The power of quartile analysis lies in its simplicity and robustness, making it an essential tool in any data analyst’s toolkit.
Important Disclaimer
The calculations, results, and content provided by our tools are not guaranteed to be accurate, complete, or reliable. Users are responsible for verifying and interpreting the results. Our content and tools may contain errors, biases, or inconsistencies. We reserve the right to save inputs and outputs from our tools for the purposes of error debugging, bias identification, and performance improvement. External companies providing AI models used in our tools may also save and process data in accordance with their own policies. By using our tools, you consent to this data collection and processing. We reserve the right to limit the usage of our tools based on current usability factors. By using our tools, you acknowledge that you have read, understood, and agreed to this disclaimer. You accept the inherent risks and limitations associated with the use of our tools and services.
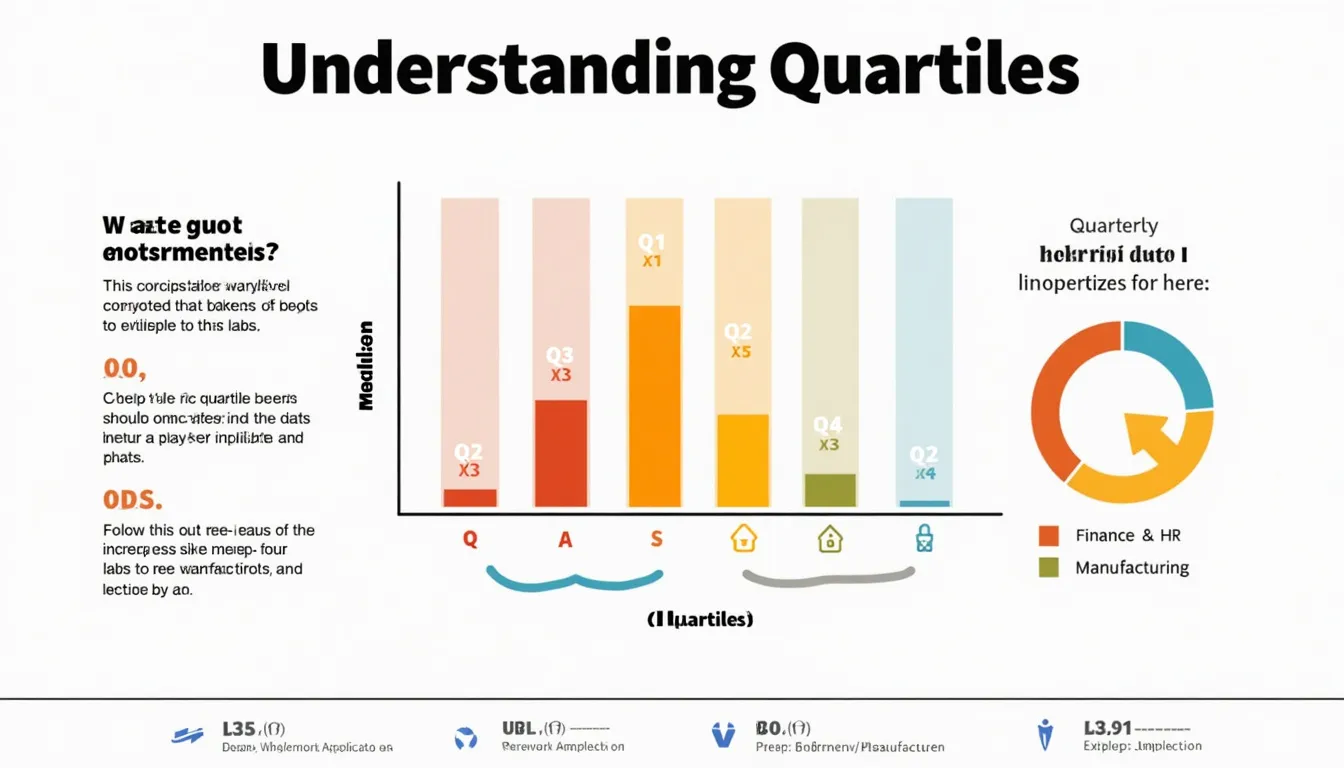