How to Use the Z-Statistic Calculator Effectively
Our Z-Statistic Calculator is a powerful tool designed to help you perform one-sample z-tests for both means and proportions. Follow these steps to use the calculator effectively:
Step 1: Select the Type of Test
Choose between two options:
- One-sample z-test for means: Use this when comparing a sample mean to a known population mean.
- One-sample z-test for proportions: Use this when comparing a sample proportion to a known population proportion.
Step 2: Choose the Direction of the Test
Select one of the following:
- Two-tailed: Use when you want to test for any significant difference in either direction.
- One-tailed (left): Use when you’re testing if the sample statistic is significantly less than the population parameter.
- One-tailed (right): Use when you’re testing if the sample statistic is significantly greater than the population parameter.
Step 3: Enter the Significance Level
Input the desired significance level (α). Common values include:
- 0.10 (10% significance level)
- 0.05 (5% significance level)
- 0.01 (1% significance level)
Step 4: Input Test-Specific Values
Depending on the type of test you selected, enter the following information:
For One-sample z-test for means:
- Sample Mean (x̄): Enter the mean of your sample data (e.g., 105).
- Population Mean (μ): Enter the known or hypothesized population mean (e.g., 100).
- Population Standard Deviation (σ): Enter the known standard deviation of the population (e.g., 15).
- Sample Size (n): Enter the number of observations in your sample (e.g., 25).
For One-sample z-test for proportions:
- Sample Proportion (p̂): Enter the proportion from your sample data (e.g., 0.6).
- Population Proportion (p): Enter the known or hypothesized population proportion (e.g., 0.5).
- Sample Size (n): Enter the number of observations in your sample (e.g., 100).
Step 5: Calculate and Interpret Results
Click the “Calculate” button to generate the results. The calculator will display:
- Z-Statistic
- P-value
- Critical Value(s)
- Conclusion (whether to reject or fail to reject the null hypothesis)
- A graphical representation of the z-distribution with marked critical regions and z-statistic
Understanding Z-Statistics: Definition, Purpose, and Benefits
The z-statistic is a fundamental concept in statistical analysis, particularly in hypothesis testing and inferential statistics. It measures how many standard deviations away a sample statistic is from its hypothesized population parameter.
Definition of Z-Statistic
A z-statistic (or z-score) is a standardized measure that quantifies the difference between an observed value and the mean of a distribution in units of standard deviations. For a normal distribution, the z-statistic follows a standard normal distribution with a mean of 0 and a standard deviation of 1.
Purpose of Z-Statistics
The primary purposes of z-statistics include:
- Hypothesis testing for population means and proportions
- Constructing confidence intervals
- Comparing different distributions
- Identifying outliers in a dataset
- Standardizing scores from different distributions
Benefits of Using Z-Statistics
Utilizing z-statistics in your statistical analyses offers several advantages:
- Standardization: Z-statistics allow for comparison across different normal distributions by converting them to a standard scale.
- Probability interpretation: Z-scores directly correspond to probabilities in the standard normal distribution, facilitating easy interpretation of results.
- Versatility: Z-statistics can be applied to various types of data and research questions, making them a versatile tool in statistics.
- Simplicity: The concept of z-scores is relatively straightforward, making it accessible to researchers with varying levels of statistical expertise.
- Foundation for advanced techniques: Understanding z-statistics provides a solid foundation for more complex statistical methods and analyses.
How the Z-Statistic Calculator Addresses User Needs
Our Z-Statistic Calculator is designed to address various user needs and solve specific problems in statistical analysis. Here’s how it caters to different requirements:
1. Streamlining Hypothesis Testing
The calculator automates the process of hypothesis testing, saving time and reducing the likelihood of calculation errors. Users can quickly obtain results for both one-sample z-tests for means and proportions without the need for manual calculations.
2. Flexible Test Directions
By offering options for two-tailed, left-tailed, and right-tailed tests, the calculator accommodates various research questions and hypotheses. This flexibility allows users to tailor their analysis to their specific needs.
3. Comprehensive Results
The calculator provides a complete set of results, including the z-statistic, p-value, critical value(s), and a clear conclusion. This comprehensive output helps users make informed decisions about their hypotheses without the need for additional calculations or reference tables.
4. Visual Representation
The inclusion of a graphical representation of the z-distribution helps users visualize their results in context. This visual aid enhances understanding of the statistical significance and the relationship between the calculated z-statistic and the critical regions.
5. Educational Tool
For students and those learning statistics, the calculator serves as an educational tool. By providing step-by-step results, it helps users understand the relationship between inputs (such as sample statistics and population parameters) and the resulting z-statistic and p-value.
6. Accessibility
The web-based nature of the calculator makes it accessible to users across different devices and platforms, without the need for specialized statistical software.
Example Calculation: One-sample Z-Test for Means
Let’s walk through an example calculation to illustrate how the calculator solves a specific problem:
Suppose a company claims that their energy drink increases reaction time by an average of 50 milliseconds. A researcher wants to test this claim and collects the following data:
- Sample Mean (x̄) = 53 ms
- Population Mean (μ) = 50 ms (claimed value)
- Population Standard Deviation (σ) = 8 ms (known from previous studies)
- Sample Size (n) = 36
- Significance Level (α) = 0.05
- Test Direction: Two-tailed
Using these inputs, the calculator would perform the following steps:
Step 1: Calculate the Z-Statistic
$$ z = \frac{\bar{x} – \mu}{\sigma / \sqrt{n}} = \frac{53 – 50}{8 / \sqrt{36}} = \frac{3}{1.333} = 2.25 $$Step 2: Calculate the P-value
For a two-tailed test, the p-value is calculated as:
$$ p-value = 2 \times P(Z > |2.25|) = 2 \times 0.0122 = 0.0244 $$Step 3: Determine the Critical Value
For a two-tailed test with α = 0.05, the critical values are ±1.96.
Step 4: Draw a Conclusion
Since |2.25| > 1.96 and 0.0244 < 0.05, we reject the null hypothesis.
The calculator would display these results, along with the conclusion: “Reject the null hypothesis. There is sufficient evidence to suggest that the true mean reaction time increase differs from the claimed 50 ms.”
Practical Applications of the Z-Statistic Calculator
The Z-Statistic Calculator has a wide range of practical applications across various fields. Here are some examples illustrating its versatility:
1. Quality Control in Manufacturing
A manufacturing company can use the z-test for means to check if their production line is meeting specifications. For instance, they might test whether the average weight of packaged products differs significantly from the target weight.
Example:
- Target weight (μ) = 500 grams
- Sample mean (x̄) = 498 grams
- Known standard deviation (σ) = 5 grams
- Sample size (n) = 100
Using the calculator, they can quickly determine if the observed difference is statistically significant and decide whether adjustments to the production line are necessary.
2. Medical Research
Researchers in medicine often use z-tests to compare sample proportions to known population proportions. For example, testing whether a new treatment method significantly increases the recovery rate compared to the standard treatment.
Example:
- Known recovery rate with standard treatment (p) = 0.70
- Observed recovery rate with new treatment (p̂) = 0.78
- Sample size (n) = 200
The z-test for proportions can help determine if the new treatment shows a statistically significant improvement.
3. Market Research
Marketing professionals can use z-tests to compare survey results to industry benchmarks or previous years’ data. For instance, testing whether customer satisfaction ratings have significantly improved after implementing new service protocols.
Example:
- Industry average satisfaction score (μ) = 7.5 out of 10
- Company’s sample mean satisfaction score (x̄) = 7.8
- Known standard deviation (σ) = 1.2
- Sample size (n) = 500
The z-test can help determine if the company’s satisfaction scores are significantly higher than the industry average.
4. Educational Assessment
Educators and educational researchers can use z-tests to compare test scores of a particular class or school to national or state averages.
Example:
- National average SAT math score (μ) = 520
- School’s average SAT math score (x̄) = 535
- Known standard deviation (σ) = 100
- Number of students tested (n) = 150
The z-test can help determine if the school’s performance is significantly different from the national average.
5. Environmental Science
Environmental scientists can use z-tests to compare observed pollution levels to regulatory standards or historical averages.
Example:
- EPA standard for particulate matter (μ) = 35 μg/m³
- Observed average in a city (x̄) = 37 μg/m³
- Known standard deviation (σ) = 5 μg/m³
- Number of measurements (n) = 100
The z-test can help determine if the city’s air quality is significantly worse than the EPA standard.
Frequently Asked Questions (FAQ)
1. What is the difference between a z-test and a t-test?
The main difference lies in the population standard deviation:
- Z-test: Used when the population standard deviation is known.
- T-test: Used when the population standard deviation is unknown and must be estimated from the sample.
Additionally, t-tests are generally used for smaller sample sizes (n < 30), while z-tests are typically used for larger samples.
2. When should I use a one-tailed test versus a two-tailed test?
Choose based on your research question:
- One-tailed test: Use when you’re only interested in deviations in one direction (e.g., testing if a new drug is better than a placebo, but not if it’s worse).
- Two-tailed test: Use when you’re interested in deviations in either direction (e.g., testing if a new drug has any effect, whether positive or negative).
3. How do I interpret the p-value?
The p-value represents the probability of obtaining a test statistic as extreme as the observed one, assuming the null hypothesis is true. A smaller p-value (typically < 0.05) suggests stronger evidence against the null hypothesis.
4. What sample size is required for a z-test?
Generally, a sample size of 30 or more is considered sufficient for a z-test. However, for proportions, ensure that np ≥ 5 and n(1-p) ≥ 5, where n is the sample size and p is the proportion.
5. Can I use a z-test if my data isn’t normally distributed?
The Central Limit Theorem states that for large sample sizes (typically n ≥ 30), the sampling distribution of the mean approximates a normal distribution, regardless of the underlying population distribution. Therefore, z-tests can often be used even when the data isn’t perfectly normal, as long as the sample size is sufficiently large.
6. How do I choose the appropriate significance level?
Common significance levels are 0.05, 0.01, and 0.10. The choice depends on the field of study and the consequences of Type I errors (falsely rejecting the null hypothesis). More stringent levels (e.g., 0.01) are used when false positives are particularly costly or dangerous.
7. What’s the difference between statistical significance and practical significance?
Statistical significance (determined by the p-value) indicates whether an observed difference is likely due to chance. Practical significance considers whether the difference is large enough to be meaningful in real-world applications. A result can be statistically significant but not practically significant, especially with very large sample sizes.
8. Can I use the z-test calculator for comparing two samples?
This calculator is designed for one-sample z-tests. For comparing two samples, you would need a two-sample z-test or t-test, depending on whether the population standard deviations are known.
9. How do I report the results of a z-test in a paper or presentation?
Typically, you should report:
- The test statistic (z-value)
- The p-value
- The significance level used
- Your conclusion in plain language
For example: “A one-sample z-test revealed that the sample mean (M = 53, SD = 8) was significantly different from the hypothesized population mean of 50, z = 2.25, p = .024, α = .05.”
10. What are some common mistakes to avoid when using z-tests?
Common pitfalls include:
- Using a z-test when a t-test is more appropriate (i.e., when the population standard deviation is unknown)
- Misinterpreting the p-value as the probability that the null hypothesis is true
- Ignoring the assumptions of the test (e.g., independence of observations, normality for small samples)
- Overrelying on p-values without considering effect sizes or practical significance
- Using one-tailed tests without a strong directional hypothesis
By understanding these common questions and considerations, users can more effectively utilize the Z-Statistic Calculator and interpret its results in their statistical analyses.
Is this tool helpful?
Important Disclaimer
The calculations, results, and content provided by our tools are not guaranteed to be accurate, complete, or reliable. Users are responsible for verifying and interpreting the results. Our content and tools may contain errors, biases, or inconsistencies. We reserve the right to save inputs and outputs from our tools for the purposes of error debugging, bias identification, and performance improvement. External companies providing AI models used in our tools may also save and process data in accordance with their own policies. By using our tools, you consent to this data collection and processing. We reserve the right to limit the usage of our tools based on current usability factors. By using our tools, you acknowledge that you have read, understood, and agreed to this disclaimer. You accept the inherent risks and limitations associated with the use of our tools and services.
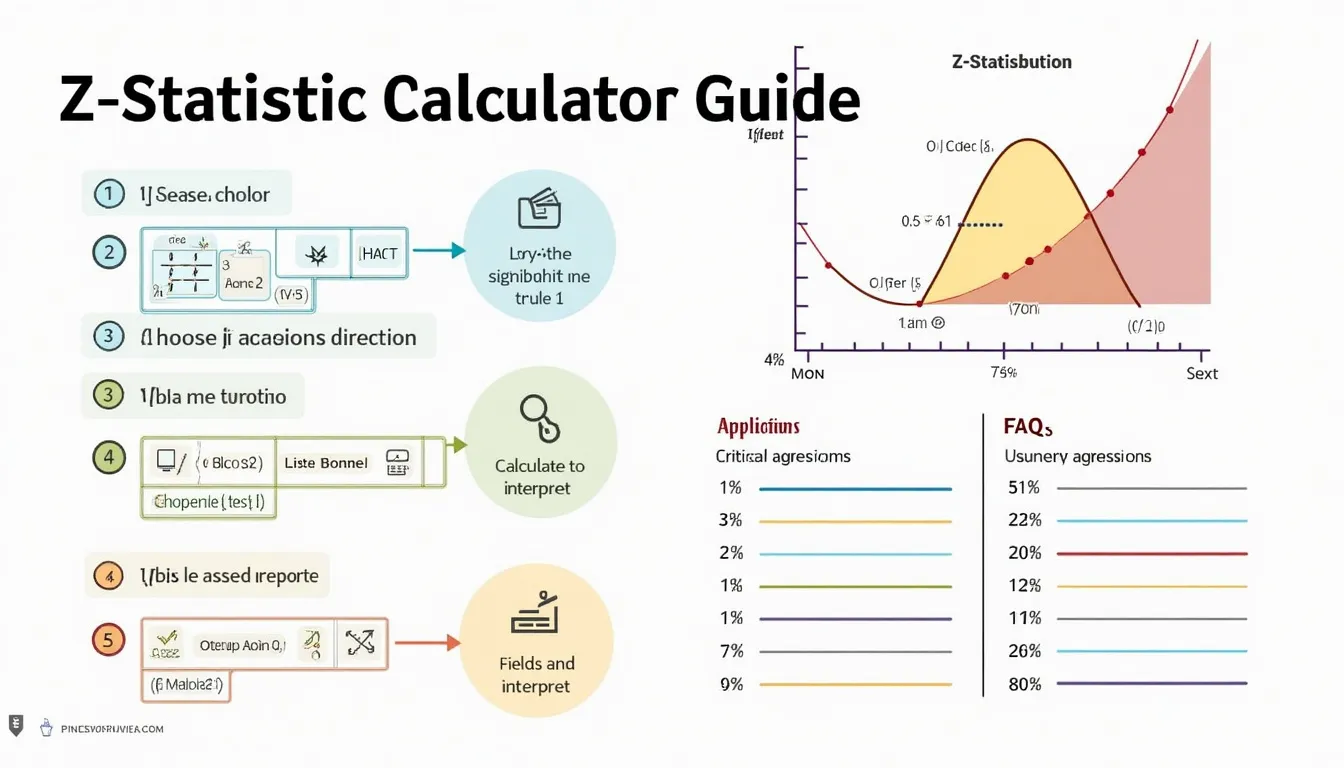